Session: ABC: Digital Analytical Sciences
Session Chair: Prof. Dr. Günter Gauglitz, Prof. Dr. Ulrich Panne
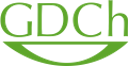
English
Digital Transformation of the Analytics Lab - A Key Factor to Drive Innovation
Joachim Richert, BASF SEAs drivers of the innovative potential of the chemical and pharmaceutical industry laboratories find themselves in the focus of the digital transformation of the industry. Design of experiment (DoE) approaches and model-based simulation leveraging digital twins of production processes shorten development cycles and time-to-market of novel products. Real-time data from process development, manufacturing, application development and supply chain allow a more sustainable management of product volume, resources and quality. Access to chemical information through on-line, in-line and at-line analytical data allow for the first time real-time optimization of production processes driven by chemistry. The digital transformation of chemistry shifts the analytical chemist and the analytical laboratory further in the center of the innovation process. [1] With the digital transformation of the analytical laboratory becoming a decisive aspect novel approaches towards a “Lab-of-the Future” are required. Some interesting “Blue Ocean” approaches have been looked at in the recent past. However, in daily life existing infra-structure and aging production plants provide difficult boundary conditions to the industry. [2] In addition, proprietary communication protocols and data formats represent additional hurdles to the collaboration of smart lab instrumentation, robotics and lab data management systems. Without a more rigorous standardization of the data infrastructure, novel approaches to software development and a wholistic view of the laboratory processes within the innovation chain a true digital transformation will not be possible. Advances based on new protocols such as OPC-UA or SiLA 2 might catalyze the digital transformation of the analytical laboratory. [3] [4]
English
Digital Transformation of the Analytics Lab - A Key Factor to Drive Innovation
Joachim Richert, BASF SEAs drivers of the innovative potential of the chemical and pharmaceutical industry laboratories find themselves in the focus of the digital transformation of the industry. Design of experiment (DoE) approaches and model-based simulation leveraging digital twins of production processes shorten development cycles and time-to-market of novel products. Real-time data from process development, manufacturing, application development and supply chain allow a more sustainable management of product volume, resources and quality. Access to chemical information through on-line, in-line and at-line analytical data allow for the first time real-time optimization of production processes driven by chemistry. The digital transformation of chemistry shifts the analytical chemist and the analytical laboratory further in the center of the innovation process. [1] With the digital transformation of the analytical laboratory becoming a decisive aspect novel approaches towards a “Lab-of-the Future” are required. Some interesting “Blue Ocean” approaches have been looked at in the recent past. However, in daily life existing infra-structure and aging production plants provide difficult boundary conditions to the industry. [2] In addition, proprietary communication protocols and data formats represent additional hurdles to the collaboration of smart lab instrumentation, robotics and lab data management systems. Without a more rigorous standardization of the data infrastructure, novel approaches to software development and a wholistic view of the laboratory processes within the innovation chain a true digital transformation will not be possible. Advances based on new protocols such as OPC-UA or SiLA 2 might catalyze the digital transformation of the analytical laboratory. [3] [4]
English
Digital Transformation of the Analytics Lab - A Key Factor to Drive Innovation
Joachim Richert, BASF SEAs drivers of the innovative potential of the chemical and pharmaceutical industry laboratories find themselves in the focus of the digital transformation of the industry. Design of experiment (DoE) approaches and model-based simulation leveraging digital twins of production processes shorten development cycles and time-to-market of novel products. Real-time data from process development, manufacturing, application development and supply chain allow a more sustainable management of product volume, resources and quality. Access to chemical information through on-line, in-line and at-line analytical data allow for the first time real-time optimization of production processes driven by chemistry. The digital transformation of chemistry shifts the analytical chemist and the analytical laboratory further in the center of the innovation process. [1] With the digital transformation of the analytical laboratory becoming a decisive aspect novel approaches towards a “Lab-of-the Future” are required. Some interesting “Blue Ocean” approaches have been looked at in the recent past. However, in daily life existing infra-structure and aging production plants provide difficult boundary conditions to the industry. [2] In addition, proprietary communication protocols and data formats represent additional hurdles to the collaboration of smart lab instrumentation, robotics and lab data management systems. Without a more rigorous standardization of the data infrastructure, novel approaches to software development and a wholistic view of the laboratory processes within the innovation chain a true digital transformation will not be possible. Advances based on new protocols such as OPC-UA or SiLA 2 might catalyze the digital transformation of the analytical laboratory. [3] [4]
English
Good vibrations: towards rapid screening of energetic materials properties
Adam A. L. Michalchuk, Bundesanstalt für Materialforschung und –prüfung (BAM)Energetic materials (explosives, propellants, gas generators, and pyrotechnics; EMs) represent an important class of functional materials. With increasing environmental pressures and safety regulations, there is growing need to develop new EMs. Arguably the most important characteristic of new EMs is their sensitivity to initiation. BAM has long been at the forefront of technological developments aimed at assessing these safety parameters. Currently, standard approaches require the synthesis and testing of new EMs; often at great risk to personnel safety. We will discuss a new model for rapidly screening EM sensitivity based on in silico generated data. Moreover, we will discuss the extension of this model towards predictions from rapidly attainable experimental data, and hence its potential as a screening tool to direct the experimental preparation of new EMs.
English
Good vibrations: towards rapid screening of energetic materials properties
Adam A. L. Michalchuk, Bundesanstalt für Materialforschung und –prüfung (BAM)Energetic materials (explosives, propellants, gas generators, and pyrotechnics; EMs) represent an important class of functional materials. With increasing environmental pressures and safety regulations, there is growing need to develop new EMs. Arguably the most important characteristic of new EMs is their sensitivity to initiation. BAM has long been at the forefront of technological developments aimed at assessing these safety parameters. Currently, standard approaches require the synthesis and testing of new EMs; often at great risk to personnel safety. We will discuss a new model for rapidly screening EM sensitivity based on in silico generated data. Moreover, we will discuss the extension of this model towards predictions from rapidly attainable experimental data, and hence its potential as a screening tool to direct the experimental preparation of new EMs.
English
Good vibrations: towards rapid screening of energetic materials properties
Adam A. L. Michalchuk, Bundesanstalt für Materialforschung und –prüfung (BAM)Energetic materials (explosives, propellants, gas generators, and pyrotechnics; EMs) represent an important class of functional materials. With increasing environmental pressures and safety regulations, there is growing need to develop new EMs. Arguably the most important characteristic of new EMs is their sensitivity to initiation. BAM has long been at the forefront of technological developments aimed at assessing these safety parameters. Currently, standard approaches require the synthesis and testing of new EMs; often at great risk to personnel safety. We will discuss a new model for rapidly screening EM sensitivity based on in silico generated data. Moreover, we will discuss the extension of this model towards predictions from rapidly attainable experimental data, and hence its potential as a screening tool to direct the experimental preparation of new EMs.
English
Chemometrics or machine learning for the analysis of Raman spectroscopic related data?
Thomas Bocklitz, Leibniz Institute of Photonic Technology (IPHT)Spectroscopic imaging like Raman spectral imaging can be utilized to determine the biochemical composition of a sample in a non-destructive manner. This results from the fact the Raman spectra represents the biochemical composition of the sample and that the Raman measurements are non-destructive and label-free. Due to label-free character of spectroscopic imaging, the techniques represent an untargeted imaging approach like most of the OMICS-technologies. In order to utilize it for applications like diagnostics or analytics, machine learning methods and chemometrics are utilized, which translate the spectral measurements into higher-level information in the application context. While chemometrics is a sub-domain of analytical chemistry dealing with the application of signal processing, pattern recognition and multivariate statistical methods for chemical data, machine learning is a field in computer science. Both fields have in common that computational methods are used and models are created, which can solve a given task. Such a task might be the use of non-targeted image and spectral data like Raman spectra or spectroscopic images for diagnostic or analytic application scenarios. In this contribution the use of chemometrics and machine learning for the analysis of spectroscopic image data consisting of coherent anti-Stokes Raman spectroscopy (CARS), two-photon-excited fluorescence (TPEF) and second harmonic generation (SHG) will be presented. In order start the analysis the image data need to be standardized using uneven-illumination correction [1,2], segmentation methods [3], filtering and scaling approaches. The standardized spectroscopic image data is further analyzed using a combination of feature extraction and simple classification models (classical machine learning) [4-7] or deep learning [8-10]. In this contribution the advantages and disadvantages regarding both machine learning schemes will be shown and discussed.
English
Chemometrics or machine learning for the analysis of Raman spectroscopic related data?
Thomas Bocklitz, Leibniz Institute of Photonic Technology (IPHT)Spectroscopic imaging like Raman spectral imaging can be utilized to determine the biochemical composition of a sample in a non-destructive manner. This results from the fact the Raman spectra represents the biochemical composition of the sample and that the Raman measurements are non-destructive and label-free. Due to label-free character of spectroscopic imaging, the techniques represent an untargeted imaging approach like most of the OMICS-technologies. In order to utilize it for applications like diagnostics or analytics, machine learning methods and chemometrics are utilized, which translate the spectral measurements into higher-level information in the application context. While chemometrics is a sub-domain of analytical chemistry dealing with the application of signal processing, pattern recognition and multivariate statistical methods for chemical data, machine learning is a field in computer science. Both fields have in common that computational methods are used and models are created, which can solve a given task. Such a task might be the use of non-targeted image and spectral data like Raman spectra or spectroscopic images for diagnostic or analytic application scenarios. In this contribution the use of chemometrics and machine learning for the analysis of spectroscopic image data consisting of coherent anti-Stokes Raman spectroscopy (CARS), two-photon-excited fluorescence (TPEF) and second harmonic generation (SHG) will be presented. In order start the analysis the image data need to be standardized using uneven-illumination correction [1,2], segmentation methods [3], filtering and scaling approaches. The standardized spectroscopic image data is further analyzed using a combination of feature extraction and simple classification models (classical machine learning) [4-7] or deep learning [8-10]. In this contribution the advantages and disadvantages regarding both machine learning schemes will be shown and discussed.
English
Chemometrics or machine learning for the analysis of Raman spectroscopic related data?
Thomas Bocklitz, Leibniz Institute of Photonic Technology (IPHT)Spectroscopic imaging like Raman spectral imaging can be utilized to determine the biochemical composition of a sample in a non-destructive manner. This results from the fact the Raman spectra represents the biochemical composition of the sample and that the Raman measurements are non-destructive and label-free. Due to label-free character of spectroscopic imaging, the techniques represent an untargeted imaging approach like most of the OMICS-technologies. In order to utilize it for applications like diagnostics or analytics, machine learning methods and chemometrics are utilized, which translate the spectral measurements into higher-level information in the application context. While chemometrics is a sub-domain of analytical chemistry dealing with the application of signal processing, pattern recognition and multivariate statistical methods for chemical data, machine learning is a field in computer science. Both fields have in common that computational methods are used and models are created, which can solve a given task. Such a task might be the use of non-targeted image and spectral data like Raman spectra or spectroscopic images for diagnostic or analytic application scenarios. In this contribution the use of chemometrics and machine learning for the analysis of spectroscopic image data consisting of coherent anti-Stokes Raman spectroscopy (CARS), two-photon-excited fluorescence (TPEF) and second harmonic generation (SHG) will be presented. In order start the analysis the image data need to be standardized using uneven-illumination correction [1,2], segmentation methods [3], filtering and scaling approaches. The standardized spectroscopic image data is further analyzed using a combination of feature extraction and simple classification models (classical machine learning) [4-7] or deep learning [8-10]. In this contribution the advantages and disadvantages regarding both machine learning schemes will be shown and discussed.
English
Digital Detective Work: Connecting Cheminformatics, Mass Spectrometry and our Environment
Emma Schymanski, UNIVERSITY OF LUXEMBOURGThe environment and the chemicals to which we are exposed is incredibly complex, with around 100 million chemicals in the largest open chemical databases and over 70,000 in household use alone. Detectable molecules in complex samples can now be captured using high resolution mass spectrometry (HRMS), which provides a “snapshot” of all chemicals present in a sample and allows for retrospective data analysis through digital archiving. However, scientists cannot yet identify most of the tens of thousands of features in each sample, leading to critical bottlenecks in identification and data interpretation. This gives rise to the need for “digital detective work”. Unknown identification remains extremely time consuming and, in many cases, a matter of luck. Prioritizing efforts to find significant metabolites or potentially toxic substances responsible for observed biological effects are the key, which involves reconciling highly complex samples with expert knowledge and careful validation. This talk will cover European, US and worldwide community initiatives to help connect knowledge on chemistry and toxicity with environmental observations - from compound databases to spectral libraries and retrospective screening. It will touch on the challenges of standardized structure representations, data curation, deposition and communication between resources, with a focus on recent activities we have undertaken to explore empowering both PubChem (https://pubchem.ncbi.nlm.nih.gov/) and MetFrag (https://msbi.ipb-halle.de/MetFrag/) for use in exposomics studies. Finally, it will show how interdisciplinary efforts and data sharing can facilitate research in metabolomics, exposomics and beyond.
English
Digital Detective Work: Connecting Cheminformatics, Mass Spectrometry and our Environment
Emma Schymanski, UNIVERSITY OF LUXEMBOURGThe environment and the chemicals to which we are exposed is incredibly complex, with around 100 million chemicals in the largest open chemical databases and over 70,000 in household use alone. Detectable molecules in complex samples can now be captured using high resolution mass spectrometry (HRMS), which provides a “snapshot” of all chemicals present in a sample and allows for retrospective data analysis through digital archiving. However, scientists cannot yet identify most of the tens of thousands of features in each sample, leading to critical bottlenecks in identification and data interpretation. This gives rise to the need for “digital detective work”. Unknown identification remains extremely time consuming and, in many cases, a matter of luck. Prioritizing efforts to find significant metabolites or potentially toxic substances responsible for observed biological effects are the key, which involves reconciling highly complex samples with expert knowledge and careful validation. This talk will cover European, US and worldwide community initiatives to help connect knowledge on chemistry and toxicity with environmental observations - from compound databases to spectral libraries and retrospective screening. It will touch on the challenges of standardized structure representations, data curation, deposition and communication between resources, with a focus on recent activities we have undertaken to explore empowering both PubChem (https://pubchem.ncbi.nlm.nih.gov/) and MetFrag (https://msbi.ipb-halle.de/MetFrag/) for use in exposomics studies. Finally, it will show how interdisciplinary efforts and data sharing can facilitate research in metabolomics, exposomics and beyond.
English
Digital Detective Work: Connecting Cheminformatics, Mass Spectrometry and our Environment
Emma Schymanski, UNIVERSITY OF LUXEMBOURGThe environment and the chemicals to which we are exposed is incredibly complex, with around 100 million chemicals in the largest open chemical databases and over 70,000 in household use alone. Detectable molecules in complex samples can now be captured using high resolution mass spectrometry (HRMS), which provides a “snapshot” of all chemicals present in a sample and allows for retrospective data analysis through digital archiving. However, scientists cannot yet identify most of the tens of thousands of features in each sample, leading to critical bottlenecks in identification and data interpretation. This gives rise to the need for “digital detective work”. Unknown identification remains extremely time consuming and, in many cases, a matter of luck. Prioritizing efforts to find significant metabolites or potentially toxic substances responsible for observed biological effects are the key, which involves reconciling highly complex samples with expert knowledge and careful validation. This talk will cover European, US and worldwide community initiatives to help connect knowledge on chemistry and toxicity with environmental observations - from compound databases to spectral libraries and retrospective screening. It will touch on the challenges of standardized structure representations, data curation, deposition and communication between resources, with a focus on recent activities we have undertaken to explore empowering both PubChem (https://pubchem.ncbi.nlm.nih.gov/) and MetFrag (https://msbi.ipb-halle.de/MetFrag/) for use in exposomics studies. Finally, it will show how interdisciplinary efforts and data sharing can facilitate research in metabolomics, exposomics and beyond.